… using Stan & HMC
Here, I sample from an Ising-like model (I treat the random variables as continuous, between -1 and 1 and add a term to the pseudo-likelihood that resembles a beta log density).
functions {
real log_p(matrix m, real T, real alpha) {
int n = rows(m);
return( (1/T) * sum(m[2:(n-1), 2:(n-1)] .* m[1:(n-2), 2:(n-1)] +
m[2:(n-1), 2:(n-1)] .* m[2:(n-1), 1:(n-2)] +
m[2:(n-1), 2:(n-1)] .* m[3:n , 2:(n-1)] +
m[2:(n-1), 2:(n-1)] .* m[2:(n-1), 3:n ]) +
sum( log(m/2 + 0.5)*(alpha - 1) + log(0.5 - m/2)*(alpha - 1) ));
}
}
data {
int n;
real T;
real alpha;
}
parameters {
matrix<lower = -1, upper = 1>[n, n] m;
}
model {
target += log_p(m, T, alpha);
}
The matrix terms are essentially a vectorised product-sum of nearest neighbour spins.
The burn-in:
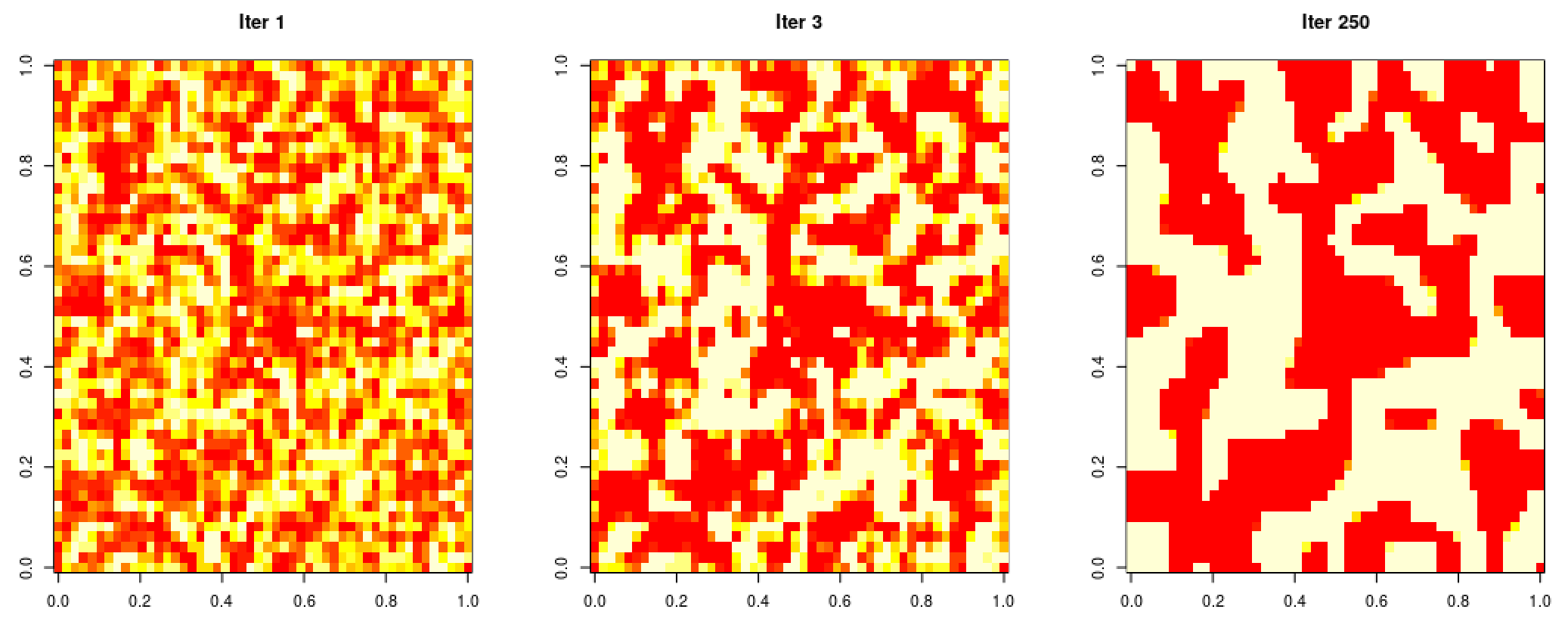
2021
Efficient Gaussian Process Computation
Using einsum for vectorizing matrix ops
Gaussian Processes in MGCV
I lay out the canonical GP interpretation of MGCV’s GAM parameters here. Prof. Wood updated the package with stationary GP smooths after a request. Running through the predict.gam
source code in a debugger, the computation of predictions appears to be as follows:
Short Side Projects
Snowflake GP
Photogrammetry
I wanted to see how easy it was to do photogrammetry (create 3d models using photos) using PyTorch3D by Facebook AI Research.
Dead Code & Syntax Trees
This post was motivated by some R code that I came across (over a thousand lines of it) with a bunch of if-statements that were never called. I wanted an automatic way to get a minimal reproducing example of a test from this file. While reading about how to do this, I came across Dead Code Elimination, which kills unused and unreachable code and variables as an example.
2020
Astrophotography
I used to do a fair bit of astrophotography in university - it’s harder to find good skies now living in the city. Here are some of my old pictures. I’ve kept making rookie mistakes (too much ISO, not much exposure time, using a slow lens, bad stacking, …), for that I apologize!
Probabilistic PCA
I’ve been reading about PPCA, and this post summarizes my understanding of it. I took a lot of this from Pattern Recognition and Machine Learning by Bishop.
Spotify Data Exploration
The main objective of this post was just to write about my typical workflow and views. The structure of this data is also outside my immediate domain so I thought it’d be fun to write up a small diary working with the data.
Random Stuff
For dealing with road/city networks, refer to Geoff Boeing’s blog and his amazing python package OSMnx. Go to Shapely for manipulation of line segments and other objects in python, networkx for networks in python and igraph for networks in R.
Morphing with GPs
The main aim here was to morph space inside a square but such that the transformation preserves some kind of ordering of the points. I wanted to use it to generate some random graphs on a flat surface and introduce spatial deformation to make the graphs more interesting.
SEIR Models
The model is described on the Compartmental Models Wikipedia Page.
Speech Synthesis
The initial aim here was to model speech samples as realizations of a Gaussian process with some appropriate covariance function, by conditioning on the spectrogram. I fit a spectral mixture kernel to segments of audio data and concatenated the segments to obtain the full waveform.
Sparse Gaussian Process Example
Minimal Working Example
2019
An Ising-Like Model
… using Stan & HMC
Stochastic Bernoulli Probabilities
Consider: